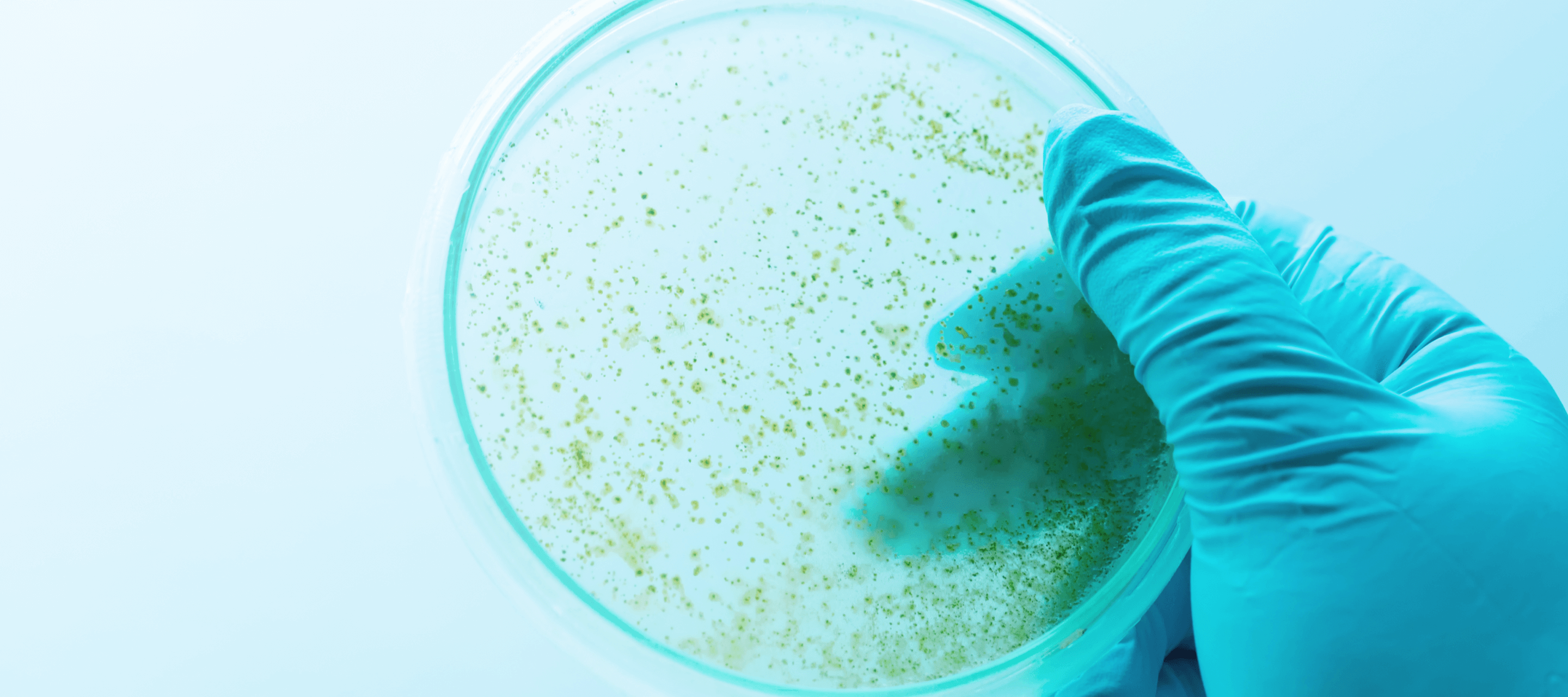
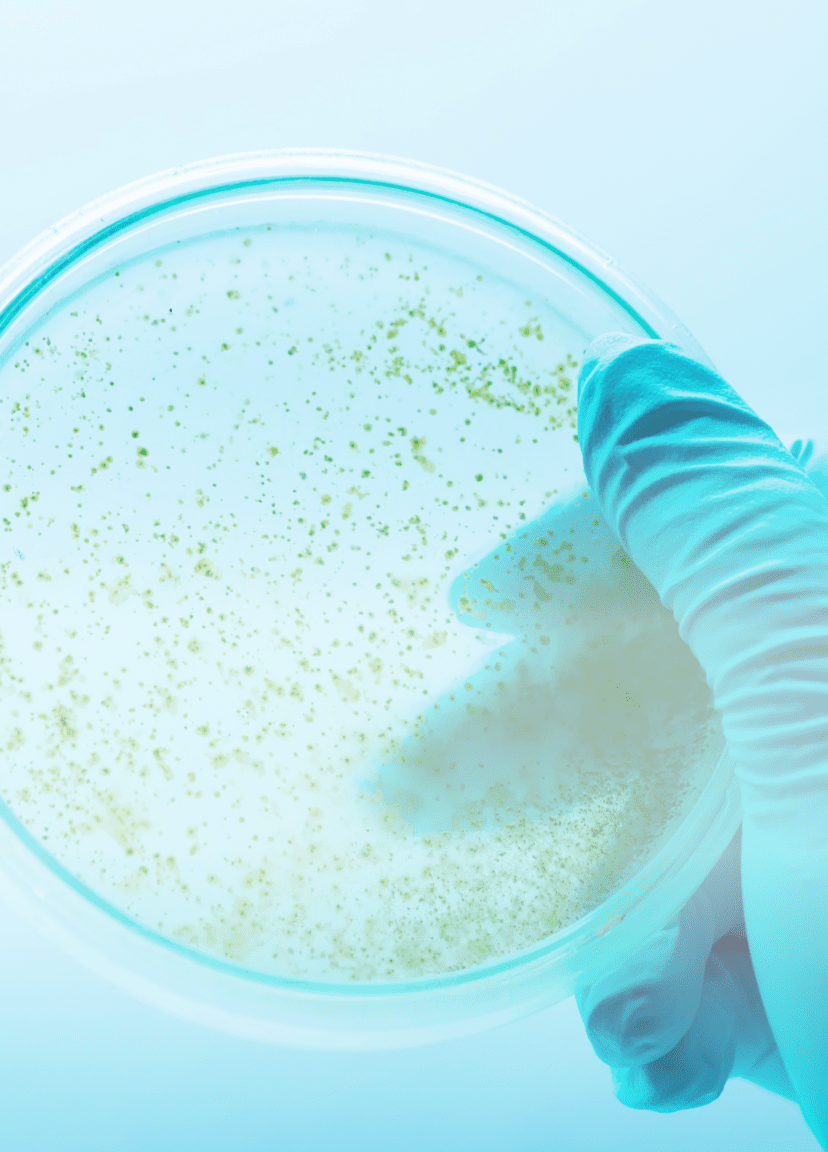
Colony counting in a clonogenic assay: The cost of human error
Manual colony counting is repetitive and error-prone, but, with automation, it can be conducted faster and more accurately
There are some techniques in the life sciences laboratory that have stubbornly remained as tedious, multistep, manual tasks, despite the increasing use of automation in the lab. Colony counting as part of a clonogenic assay is one such technique. In this article, we summarise what cell culture colony counting can tell us, and how the manual technique is usually conducted, followed by the reasons for automating this method, and how we might do so.
What is colony counting in a clonogenic assay?
In tissue culture, one of the most important pieces of information that the clonogenic assay can give us is an idea of the reproductive health of a cell. To form a colony, a cell needs to survive any treatment that we have given them, and then successfully divide into viable daughter cells in the medium we choose to grow them in. As a simple example, cells might be treated with a drug, and after some time, the fraction of cells that remain viable are determined by counting the colonies that have formed. A more complex example might be to generate two cell lines, one with a mutation in the ABC gene, and then to determine whether the mutation enables the cells to overcome inhibition of a specific biochemical pathway to survive and reproduce.
Traditionally, cells are seeded onto 10 cm tissue culture plates at a very low density (to ensure that individual colonies can be distinguished), and then incubated until surviving cells have produced colonies of more than 50 cells. A colony with over 50 cells is generally visible to the naked eye, particularly after staining the live cells with a dye like methylene blue. A digital colony counter is sometimes used to help remember which colonies have been counted and what the running total number is, by beeping and incrementing a digital counter when a scientist identifies a colony with a press of a marker pen. Because so few cells can be seeded on each plate, large numbers of plates are needed to ensure that results are reliable, and this makes manual colony counting both time consuming and error prone.
What is the impact of errors in colony counting?
Counting colonies manually means that the data generated can be subjective, and human error can occur if a scientist is distracted, tired, or in a rush. Unlike machines, humans are susceptible to limitations in terms of attention, perception, memory, and logical reasoning1. Errors such as slips, lapses, and mistakes can happen to even the most experienced and well-trained scientist2.
Errors occurring during the colony counting process can result in unreliable data, meaning that the results from one experiment cannot confidently be compared to another in the same lab, or to experimental results from other research labs. If three scientists performing colony counting on the same experiment can come up with figures, then the margin of error in the data can be substantial.
The greater the standard deviation of any data point, the less power any statistical comparisons will have. This means that it can be difficult to use data with a high degree of variability to make robust conclusions about the statistical difference between two experiments, or the significance of any such difference.
Scientific results commonly require statistical significance to have an impact on the wider research community, which means that a lab using colony counting methods which are subject to human error are less likely to be able to publish their data successfully than labs using more reliable, reproducible methods.
Automation in colony counting
Although attempts have been made to introduce automated image-based procedures to count colonies, few are able to address all the potential issues. Challenges include uniform lighting conditions, background removal, separation of merging colonies, artefact exclusion, rapid calibration, user-friendliness, and cost3,4. Commercially available tools for colony counting can be expensive, which means that laboratories often turn to open-source software such as NICE (NIST’s Integrated Colony Enumerator), CHiTA (circular Hough image transform algorithm), OpenCFU, Cell Profiler, or ImageJ3. However, open-source software can sometimes have a poorly defined development process, and it can be difficult to rely on the accuracy of an automated process if it is unclear how robustly it has been tested.
Therefore, there remains a need for automated, accurate, reproducible, fast cell-counting technologies to improve the clonogenic assay and to enable high-throughput screening in life science laboratories. Such technologies have the potential to improve the speed and accuracy of colony counting, and free scientists to focus on more rewarding, cognitive work.
Learn about Automata’s flexible, modular automated colony counting solution.
References
- Parliamentary Office of Science and Technology. Managing human error. Postnote 2001:156
- Leadership and Worker Engagement Forum. Leadership and worker involvement toolkit: Understanding human failure. Available at: https://www.hse.gov.uk/construction/lwit/assets/downloads/human-failure.pdf (last accessed February 2022)
- Siragusa M, et al. Cell colony counter called CoCoNut. PLoS ONE 2018;13:e0205823
- Choudrhy P. High-throughput method for automated colony and cell counting by digital image analysis based on edge detection. PLoS ONE 2016;11:e0148469
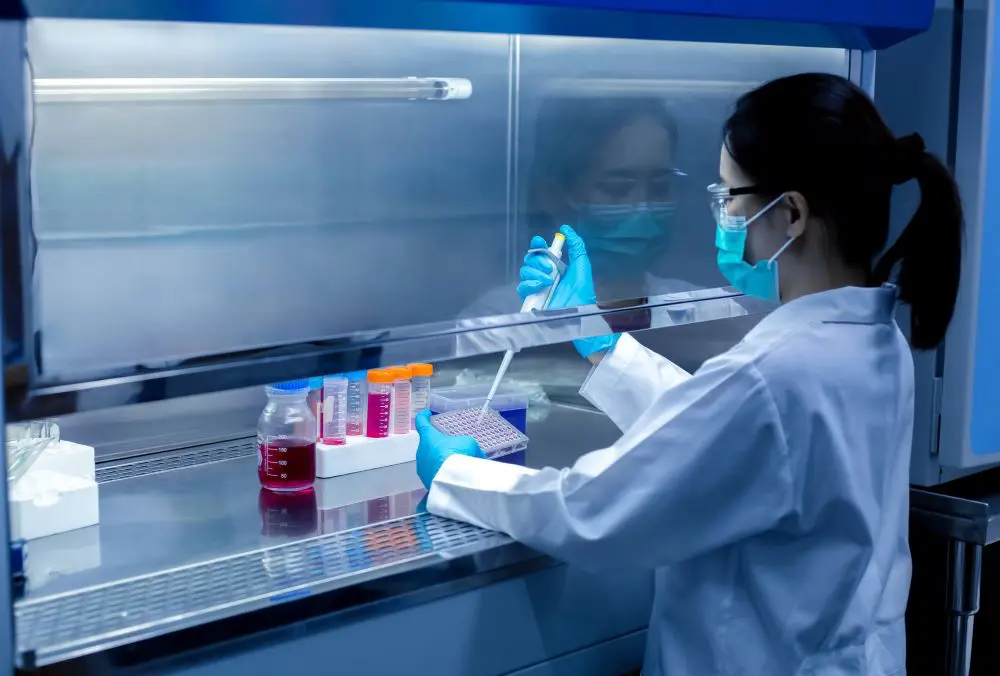
Discover the frequent mistakes made during manual ELISA assays and how automation…
Read more Common errors in manual ELISA assays and how automation can help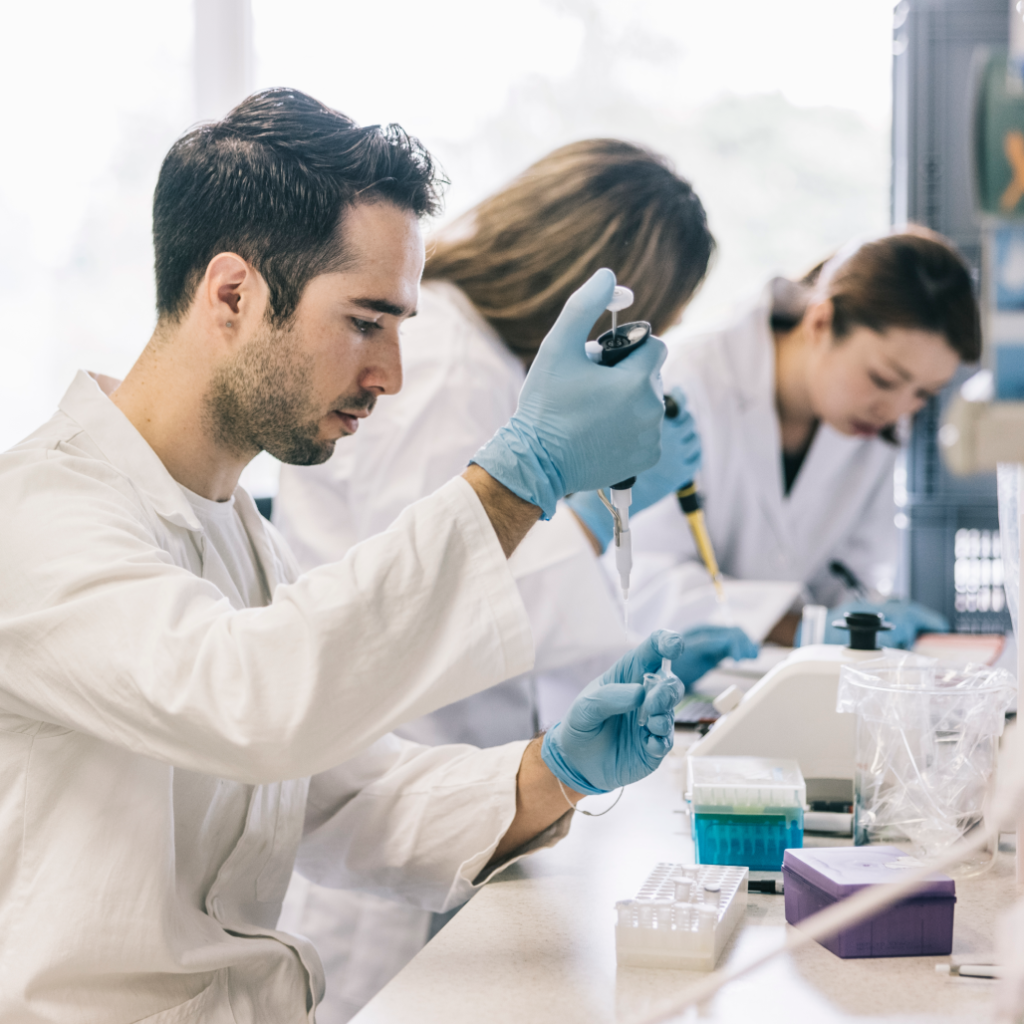
We look at what causes errors in biology lab experiments and how…
Read more Common sources of error in biology lab experiments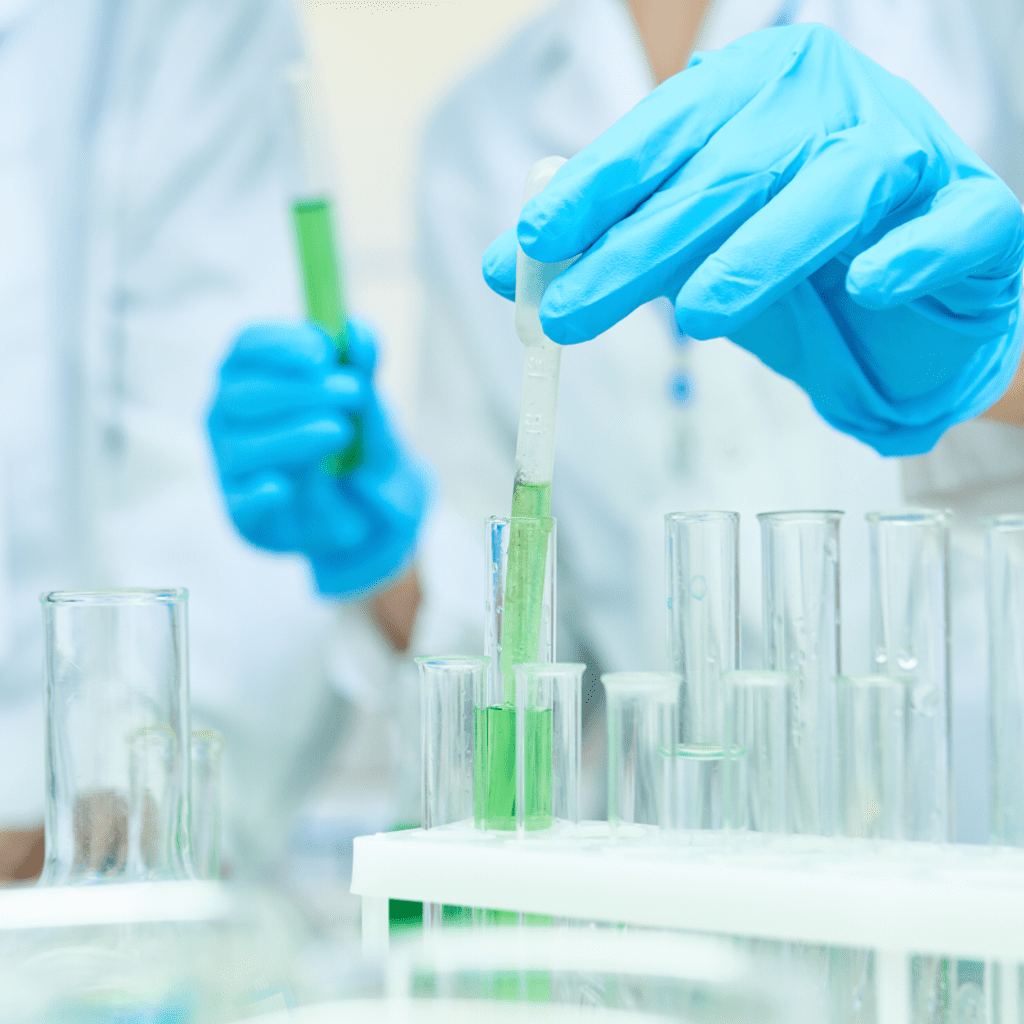
Despite protections, human error in labs still leads to incorrect diagnostics and…
Read more How automation prevents human error in the laboratory