Jump to:
– Watch the recording
– Themes at a glance
– Questions in depth
At our recent event, Advances in bio-automation, held in collaboration with Nucleate and Bits in Bio, experts from a diverse array of scientific fields gathered to network and hear from a panel of biotech innovators on some of the groundbreaking work lab automation is facilitating in their companies and beyond.
Thank you to our panellists, our partners at Nucleate and Bits in Bio, and everyone who joined us either in person or online. Below is a recording of the panel session along with a transcript of the questions and answers. To be the first to receive expert insight on lab automation and to keep up to date with our events schedule, subscribe to our newsletter.
Panel recording
Themes at a glance
The panel discussion covered several key themes surrounding the integration and challenges of automation in synthetic biology and biotechnology. A significant focus was on the importance of standardisation prior to automation. Participants highlighted that the misconception of automation as a standalone solution is common, but successful implementation requires careful change management and the alignment of existing manual processes with automated systems. This involves setting clear goals, starting with simple tasks, and gradually scaling up as more complex processes are optimised.
Another central theme was the interplay between flexibility and robustness in automation systems. The panellists discussed balancing these aspects to ensure reliable data outputs while maintaining system adaptability. They emphasised the importance of interdisciplinary collaboration, particularly between automation engineers, software developers, and biologists, to drive innovation and achieve effective automated solutions. This convergence of expertise allows for a holistic approach to problem-solving and enhances the overall efficiency and effectiveness of the automation processes being developed.
The event also showcased Nucleate’s efforts in supporting early-stage biotech founders through its Activator program. This program provides mentorship, resources, and funding to help scientists transition from lab research to startup formation. With a strong emphasis on community building and open science, Nucleate aims to democratise access to vital information and support the next generation of biotech entrepreneurs.
Data, flexibility and scalability
Automation enables efficient handling of large volumes of data, which is essential for scaling up research and production. However, it also presents challenges in terms of data integration and management.
Panellists emphasised the importance of standardising protocols before integrating automation and reiterated the importance of automation generating accurate and reliable data through validation, continuous monitoring, and dynamic error correction.
The panel agreed that a strong focus on designing automation systems that balance flexibility with robustness is necessary for automation to be more widely adopted.
Implementation and collaboration
There was an overall recognition that the initial implementation of automation can be resource-intensive. It was suggested that companies should assess their stage of development to ensure that investment in automation aligns with their current needs and future goals. There was also a recognition that successful automation projects require collaboration between various experts, including biologists, engineers, and software developers, and that this interdisciplinary approach helps design comprehensive solutions that address all aspects of the workflow.
Panellists advised starting with simple automation tasks and gradually scaling to more complex processes. This phased approach allows for troubleshooting and refining at each stage, ensuring a smooth transition. Panellists drew parallels from software development, discussing the concept of having separate production and development environments; development environments for experimentation and optimisation before automation is rolled out to production environments ready to handle standardised, validated processes.
Discussion in depth
We asked our panel a series of questions during the event, and the answers were fascinating to hear, with a multitude of perspectives offered. Read some of the insight shared below, or watch the recording, which will be available shortly.
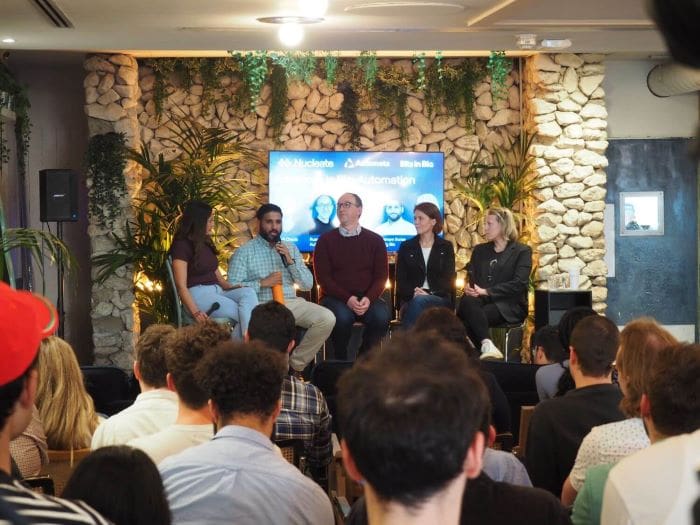
Skip to specific questions
8: What sorts of foundational changes or advancements do you think might need to happen, maybe on the hardware side or maybe at the intersection of the hardware and the software? And in general, where do you see the field of bio-automation going? What applications do you think might be untapped so far?
9: How far off do you think we are from the general goal of personalised medicine and the ability to have hyper-specific, genetically, genotype-specific drugs and therapeutics overall?
1. Can you cite a specific innovation in your company that automation has enabled, as well as a challenge you’ve encountered in implementing automation in your processes?
Satnam: An innovation that we’re super proud of, given the timing of how long we’ve been around, is being able to have a productionised experimental workflow around generating reproducible data ready for AI.
The thing with, say, liquid handling, for instance, is that you can write the code yourself, but you’ll still introduce errors. One of the things that we did was to write some code generation tools that allow the scientists to say ‘hey, these are the parameters of my experiment’ and it’ll generate the code itself. This means that 1) we have a log of every experiment that we’ve ever done, 2) the scientists don’t need to waste their time doing coding and 3) all of that data lineage – all the way back from the data and engineered cells coming out of our pipeline all the way back to the code that was used to execute that – is all tracked, is all there.
In terms of challenges, I think you need to walk before you can run. You really need to know your experiments. If you know that they’re going to change over time, know that an investment might not be the best one. If you actually have simple workflows, then you don’t need to go and spend a million dollars on a full set of kit. Understanding the fit for purpose for your stage, especially as a startup, is super important so that’s the guidance I would give from having been on the cold face.
Maureen: One of the projects that I had the opportunity to work on last summer, right around this time of year, was we developed what we believe is the world’s largest phenomics foundation model, Phenom 1. We also have Phenom Beta hosted externally for users. One of the things that automation had to enable there was just the scale of data generation. That model is trained on about 100 million images that we produced in-house, which means that over the last few years, our lab has been scaling up in just crazy ways. I think the number I heard was, in 2017 we were doing about 32 384 well plates a week and right now we’re doing about 1,500 36 well plates a week. So it’s massive scale, and that’s just on the phenomics side.
We’ve also added transcriptomics data, Invivo mix data, and some digital chemistry labs. That scale and variation of data types have meant that we’ve had a lot of challenges around things like moving data between our different compute ecosystems or from the microscope to our own supercomputer, BioHive-1 (Biohive-2 just came online a couple of weeks ago).
Data movement and managing it well and streamlining it in ways that allow us to be flexible have been big challenges.
Lilly: Our process really starts with…think of it like soup. We want to create these soups that are unstructured with the largest amount of, in our case, T-cell receptor sequences in a pool. Then we want to find ways to deconvolve activation of the T-cells against antigens, and so a lot of our process actually is around putting it all together, sort cells and sequencing them, using all of the ability to barcode different peptides and sequence DNA – that is a way for us to get the scale from a single tube. I think where we’re really trying to go is active learning can we design experiments, run them in a very short period of time, and where we generate the data, immediately update the models and then design the next set of experiments. And so getting that loop going as tight as possible is really our challenge. Some of our early team members came from Microsoft research where they set those up. They were able to update models overnight, design the next set of experiments, run them during the day, and then get this 24-hour cycle. We’re definitely not there internally yet, but that’s an ambition – to see how quickly you can get that cycle from experimentation to computation.
Russ: There are a couple of different aspects of this from my view. I’m working in a company that’s trying to really innovate and break a modern tech stack to automation, which I think in some areas has been fairly grounded for a long for a long time. Seeing us bring to the table really modern solvers that we can now utilise to solve some of the really knotty problems about automation, that excites my internal automation tech geek side, but actually, it’s when you start seeing it being applied to things that that gets really interesting. So projects are working on anything from going from open loop to closed loop automated cycles driven by AI – this is super cool, I’d love to see it start happening more and more – right through to some of the stuff we’ve been doing with things like The Royal Marsden, where you’re seeing this huge impact in solving their challenges around scaling up their clinical genomics workflows for cancer diagnostics. That’s when it becomes very real. I can spend my whole day sitting inside thinking about solving really niche problems but then seeing it being applied is where the innovation really starts coming into play.
In terms of challenges, I think it’s really interesting being a provider of automation to this. We see quite often people assuming that automation is going to solve standardisation problems, but it speaks to what other panelists were saying: what people need to start thinking about early on is how do I standardise, what I want to do to bring it to automation, and meet in the middle? I think that’s one of the most challenging areas of conversation we have.
We’ve got an incredible team that focuses on bringing manual workflows to the automated world. However, that involves an awful lot of quite complex discussions about how processes being followed now, are probably not being automated in exactly the way you want them to be, so how do you change and optimise that to bring it to a place that allows you to scale up. It’s not so much the automation that creates the challenges as the change management—the approach you take to bring those changes into an environment effectively.
Top
2: How do you strike this balance between the flexibility of the system that you’re aiming to build, and the robustness or the reliability of the data output? What are some frameworks or tips that you might suggest people think about?
Russ: Start simple and set goals. Saying you just want to automate a thing won’t get you very far. You have to think about why you want to automate it. To what end? What is the goal? What are my outcomes?
[That future-thinking will help you make some good decisions about your first step in automation, but to drive extra value from whatever you implement, you need to work with a group of automation experts to determine where and how to start.]
Satnam: We start by conceptualising using production experiments as version control, validating results against the manual version – e.g. workflow. We’ve taken a lot of inspiration from how you build software where you have production and development. Development is where flexibility happens and there’s a change process that happens to validate that because ultimately you don’t want to spend time in having the wrong thing automated, or generating garbage results quicker than you could do by hand.
Stepping back and talking about what it is that you actually want to do and working through first principles…what you can find is that you’re the bottleneck, your rate is limiting, so if you reconceptualise what you’re trying to do and can pair the automation specialist with your scientist, then you can find that you can make a lot of those processes more efficient, but when you move into production, you want to validate that. Validating against a manual workflow is probably the best way to do that. That’s the framework we use and it definitely helps.
Top
3: How much of a role do real-time monitoring and control systems play in being able to maybe iterate very quickly or have a quick development loop? Do you implement any real-time monitoring systems? How important are those to both of you?
Maureen: Yes, we do a lot of monitoring in our lab. We do have humans involved, but they’re not doing the majority of the monitoring. They’re looking at where the alerts and errors are coming from
One of the areas that I think is most interesting about our real-time monitoring is that we actually do automated image QC on the microscopes before images are uploaded into our systems, and so we can know if we have a focus issue or a lighting issue or a common air types, hopefully, before the plates are discarded. That way we can have a higher rate of successful experiments and be able to move more quickly or just not push data that’s not going to be good if it’s not recoverable or have a human get involved if we need to.
Top
4: Where have you found maybe unique places or really important places to integrate AI and machine learning, like data analytics tools, into your workflows? Have you seen a benefit from integrating experimental automated processes to the same end as AI and machine learning-based automation, or is there a trade-off or an interplay between the two sorts of automation?
Lilly: I think it really starts with the data ingestion process. There’s so much you can do to automate just how you handle data from the very beginning of your pipeline. In our early days, we were ingesting the world’s public data, and so we were bringing in data from many, many different studies with all sorts of different biological and technical confounders. That’s actually much harder than the data that comes out of our own systems where we can control exactly how the experiments were run and how the data was produced. Really harmonising data across different systems and different batches is where a lot of the work is – bringing data in properly and making it ready for machine learning models. A lot of the software automation and these large-scale compute and experimental systems are really a large focus for us.
Maureen: [We look at LLMs for really anything that we have a set process for. For example, we use LLMs a lot to look at the output of the data and look for novel biology as a starting spot for some of our research. We also use them for improving business processes – for example, we’ve looked at whether you could use LLMs to review licence agreements on external data sets and flag what the problems are there so that our legal team can spend less time reading all of that information.]
Russ: We, as a software development company, use LLMs on a daily basis but thinking about what can we do in the industry to help with this challenge – wet lab has this really interesting role to play. I see a piece of wet lab automation with a full software stack as the opportunity to own this holy trinity of data: what did I intend to do, what actually happened, and then the raw data from that. Packaging that up and making it available to be findable for something else to utilise.
I think right now, apart from working in partnership with other players who are trying to implement this, we aren’t necessarily developing AI ourselves, but we’re definitely facilitating a lot of the application of that through the huge amounts of data that we’re producing.
Satnam: We’ve taken an agentic view of this. There are specific AI/ML workflows at each stage of our process.
The first one is we have a massive metabolic map of all known biochemistry, and we search that using – I won’t go into the details, but Monte Carlo tree simulation and reinforcement learning – and we’re reinforcing the algorithms with our own data, and that’s a fairly specific task.
Another one is around enzyme engineering and how we can create a Bayesian optimisation workflow, as you mentioned before, in terms of suggesting taking data, then doing an experiment, and then suggesting the next round of experiments.
It’s not like one LLM will rule them all, we’re orchestrating multiple of them.
To speak to Russ’ point, I think the main thing is ensuring that your data layer, your data infrastructure is set up and built so that your experimental data, not just the raw values that come off, but all of the contextualization around that is available because that’s all the context that the algorithms require. We’ve actually spent probably more time on data engineering than we have on the actual ML at this point, but we’ve made a lot of progress on both sides.
Top
5: Why do you think that automation is integral in solving some of the individual problems that your companies are aiming to solve? What do you think things would look like if you weren’t to use automation technologies and why do you think it’s so key for the success of your groups.
Satnam: In terms of the pathway generation, we were doing this manually about seven or eight years ago, where a load of smart scientists would sit around and generate the hypothesis of the genes and the promoters and all the DNA components you want to insert into a cell so that it starts making stuff. That was weeks and weeks of work. We were reading papers, we were mining patents, and we could only actually generate probably about one in that month period. Fast forward to now, with our own data driving our own algorithms, we can generate multiple novel pathways to interesting targets in minutes. And that’s massive for us because we can test, we can benchmark against what’s known, but we can also really quickly test in greenfield space. And so that for us has been absolutely transformational.
Lilly: This isn’t something we’re doing now, but I think the attraction of it is really clear which is that ultimately what you want to do is to remove any human error from as many parts of your process as you can, to the extent that you can imagine a world where there’s a very defined protocol, it’s coded up, the instructions go to a bunch of machines which do the same thing. There will be some error rate, but it probably will be much lower than when humans do that same process again and again. We’re working with cells, so there’s always going to be some variability, but what we want to do is make sure that we’re not introducing experimental variability because there are different people in the lab one day or someone was on holiday. The world that you guys are enabling is a very, very reproducible world where the same instructions are given and the same output is very, very consistent across experiments.
Maureen: To the question of where would we be if we didn’t have automation in our business: for Recursion, at least, the pathway of what traditional pharma looks like is relatively clear, and it’s slow, and it’s long and it’s expensive, and most things that start at clinical trial fail. And so I think if we are able to tackle with automation any facet of that, make it faster, increase the success rate of things in clinical trials, really all of those facets, there are human lives at stake there and we feel that really deeply as a business. We are working on automation and focusing on the scale of data generation, but also the entire preclinical pipeline of how we can move faster because we know that there is urgency for a lot of people who have a lot of these diseases.
Russ: To build on that a little bit, I’ve always asked this question at the beginning of a lot of other talks we’ve done: why do we automate? Well, we automate to get more data of higher quality faster. It always comes back to the data thing, and what impact that has is always really interesting. We have client projects where the impact of speed to insight is absolutely phenomenal. For one client, I think we’re looking at bringing down what would otherwise have been a four-year early-stage medical research cycle to around four months. So when you bring that to the table, you start completely changing how quickly you can get to new therapies. What do we have if we don’t do it? That continued slow pace of discovery. It’s all about getting to better data faster.
Top
6: How do you see patient outcomes being directly affected by integrated automated workflows in your preclinical or even in your clinical studies? Do you think that there might be room for integrating automation in clinical trials in a way that hasn’t been conceptualised or realised yet? And when it comes to maybe other sorts of indications, how do you think that industry partners or other organisations on the bioprocessing front may be directly impacted by the incorporation of these workflows?
Maureen: As far as the direct impact on patient’s lives, we are currently in our first phase two clinical trials… and so there is a lot of space to look at how the process of clinical trials works. For example, with automation, is there a way to make your I&D filing automated, to be able to gather all that data across all of our sources? What is the data lineage, how do we know better on that traceability, how can I find everything we’ve run in our lab that uses this particular compound – those types of problems – I think there’s a lot of space for a standardisation of what an I&D filing looks like or what these different types of filings look like, and I would love to be able to work on some of those.
Lilly: We want to decode the immune system and reinvent how we understand how to detect, treat, and ultimately monitor that whole patient cycle.
We want to enable a world where you have an annual blood test, immediately sequenced live on-site, and you have results in your own app, and can see exactly what different diseases you’re in different stages of fighting. If you’re in an early stage of a disease, we should be able to profile that disease through that same blood test very, very cheaply, and then you’re into this world where if we have trained models on very, very large scale task-specific data, we have generalisable models that can understand how to create very, very bespoke treatments for you as a patient individually. In our case, that would be designing TCRs or T-cell receptors that really target the precise collection of peptides that may be mutated or in your own tumour, let’s say. How do you get that extremely fast, extremely precise, and in an extremely personalised way? People have been talking about that for years but it’s such an exciting moment to be in this space where we have the ability to combine software infrastructure, automation, machines, and just the ability to move much faster across that whole cycle.
Satnam: From my perspective the world that we want to get towards is one in which every product that we can make in a bio-based way, we will make in a bio-based way. This has massive implications on climate change and sustainability, but then also second-order advantages, too, in terms of localised production, removing loads of carbon from the supply chain and things like that. We see automation as a massive enabler of that perspective..
We’re working currently at lab scale and we have automation there, but really the valley of death for us is going to 100,000-litre biofermentation and the way in which to get to that level requires various iterations on scale. We really need to have, in terms of bioprocessing, much more accessibility to those facilities, but even better equipment to say ‘when is a continuous fermentation going to fail’, ‘when is it contaminated’, all those kinds of things.
And so you think about what are the challenges and what are you trying to get to? And then work backwards from there. Automation plays a load of key roles in that journey. And really, we’re looking to be a lot more predictive at lab and bench scale so that we can fully resource the programmes that we know are going to work at a large scale.
Russ: We talked about drug discovery in the SynBio [synthetic biology] space, so perhaps I’ll give some examples around patient diagnostics again.
We’re working with a lot of labs at the moment exploring the challenges they’ve got about… we are automated, we are using liquid handlers to drive things right now, but we’ve got these enormous ambitions to drive this incredible scale of sequencing every patient or diagnosing every single cancer we can get to. That just isn’t possible in the space that they’ve got right now. So what’s exciting to see is how we then bring that conversation about, ‘well, if you start layering in a deeper level of automation, that’s going to get you there, you can do that with the space you’ve got, with the people you’ve got.’ It does require some investment, but that becomes all of a sudden a possibility as opposed to an entertainable target. So seeing that impact in that diagnostic space is absolutely amazing.
Top
7: What challenges have you encountered with collaborating as a service provider, or when collaborating with other academic groups or industry partners? And what benefit do you think that you’ve been able to bring some of your collaborations in recent years?
Russ: It’s interesting because I don’t often think of collaborations as bringing challenges, though I’m sure they do often, more maybe that demands go in a direction you didn’t expect. But having said that, as a company that’s developing products incredibly fast, those partnerships and collaborations are absolutely invaluable.
I’d like to say we look at every customer as a potential partner, and I think that’s true, but you can pull out some specific examples, one really local to here: we started off working really closely with The Francis Crick Institute. They got one of our earlier systems, and it’s been beautiful to see. I joined Automata just after we had deployed that first system and you see that system change over time, developing and becoming something bigger and shinier and more advanced.
With my product development hat on those partnerships and collaborations are critical to driving forward a real pace of development.
Maureen: Collaborations have been a huge part of our acceleration as well. We have both scientific collaborations with Bayer and Roche Genentech, as well as more technology integrations with NVIDIA, and with Tempest and other data partners. Each one is really intentional to bring new capabilities onto our platform. So whether that’s new cell lines, new omix research types, it allows us to really fund and expand on the platform, as well as hopefully drive forward our process in other places.
I had the opportunity to work with some of our NVIDIA friends on our foundation model work and it was really awesome, since we were running on NVIDIA hardware, to really partner closely with them to understand how they would set up things like inference…and really gain that domain expertise and knowledge from the experts.
Top
8: What sorts of foundational changes or advancements do you think might need to happen, maybe on the hardware side or maybe at the intersection of the hardware and the software? And in general, where do you see the field of bio-automation going? What applications do you think might be untapped so far?
Russ: So I think we see two big areas of demand in the future. If it were two big buckets, one of them I would class as… it’s often referred to as orchestration, but I see it being a bit bigger than that. It’s about how we bring together not just the automation, but everything else in the lab, including the people, into one holistic system. That’s something very interesting for us at the moment.
The other piece which leans back into what we spoke about earlier on is I still don’t think we’ve cracked the data problem. Data standardisation has been kicked around in this industry for a long time and has not been solved yet. I think there’s a lot that we can do as an automation player to help drive that into a better place to some degree, working in partnership with our collaborators.
I think there’s more that can be done in the hardware space, but it’s more about driving efficiencies in those automated workcells. The big breakthrough in those two areas needs to be around bringing a whole lab together for infrastructure-level automation, and then solving this data problem once and for all.
Lilly: These are very standard ideas, but I think it’s quite interesting to imagine a world where if sequencing costs have come down dramatically, what if they really were almost zero? What if you could sequence the whole genome for a penny? What if you could compute as compute comes to, like asymptotically approaches zero? What new applications can get developed at a marginal cost of effectively zero for a lot of this data generation? I definitely don’t have the answers, but I think it’s really fun to imagine new detection methods that could be really, really fast and cheap, new ways to create bespoke models and therapies that are very, very precisely trained on patient-specific data. All of this is a world that is in our lifetime, I think – certainly within reach.
Satnam: The assay one is really important, and then potentially miniaturisation. The last one, I would say, maybe it’s not completely relevant here, but essentially same-day DNA synthesis. So you can get those loops because I think the biggest lag for us is it takes 2-4 weeks to get our library synthesised. So if we could do that same day and it wasn’t extortionally expensive, that would be great. So I think that’s one of those key innovations that we’re keeping an eye on, but it’s still a bit early.
Maureen: Also nothing crazy unique, but I do think the automation of a series of assays and series of steps and being able to use models to inform which directions we need to go next with this – we’ve gone down this pathway of ‘here’s the data that we know, where do we need to keep moving?’ to me is really exciting.
We talked about active learning earlier and using it to fill in high-value pieces of data into models: where are there gaps? What is missing to be able to use active learning, not just to pursue specific lines but also to fill in gaps in our data sets? This is interesting to me as well.
Top
9: How far off do you think we are from the general goal of personalised medicine and the ability to have genotype-specific drugs and therapeutics overall?
Lilly: The best example currently in the market is these neoantigen vaccines, where you have a tumour exome, you call a set of mutations, and you introduce peptides into patients where you’re trying to expand their native T-cell population. Those are all a bespoke trial: everyone is getting a different set of peptides that are personalised to them. We can do a lot like that across certainly on the T-cell side, so for us, we’re designing precise cocktails to target peptides that are presented in someone’s tumour that may not be replicable and may not be consistent across different patients.
There are two ways that you make that possible; either you have a really, really fast cycle time, so from the patient coming in to all of the data you need to understand that patient to the design of the right therapy being really, really fast; or you have trained very generalisable models. So what we’re trying to do is train models where we, in advance, train the right data so that our models really understand the law of recognition between T-cell receptors and antigens in our case, so that anything is in-silico query. You’re changing from this experiment’s fundamental and computational pipeline to just inference. As soon as I understand this patient’s sequences, I can immediately design the right space or go to the right space of my model to design the right therapy. I think we will get there. In our case, there’s just very, very limited data that people have generated in our specific problem and so we’re starting in very known areas – peptides have been very well validated – wut eventually we want to get to this generalisable world, which really opens up the precision, immediate design of treatments against specific patients.
Top
Your next and final automation platform is here
LINQ is a next-generation lab automation platform that can eliminate manual interactions and fully automate your genomic workflows, end to end. Explore how automation could revolutionise your lab’s capabilities: book an exploration call today.
Further reading
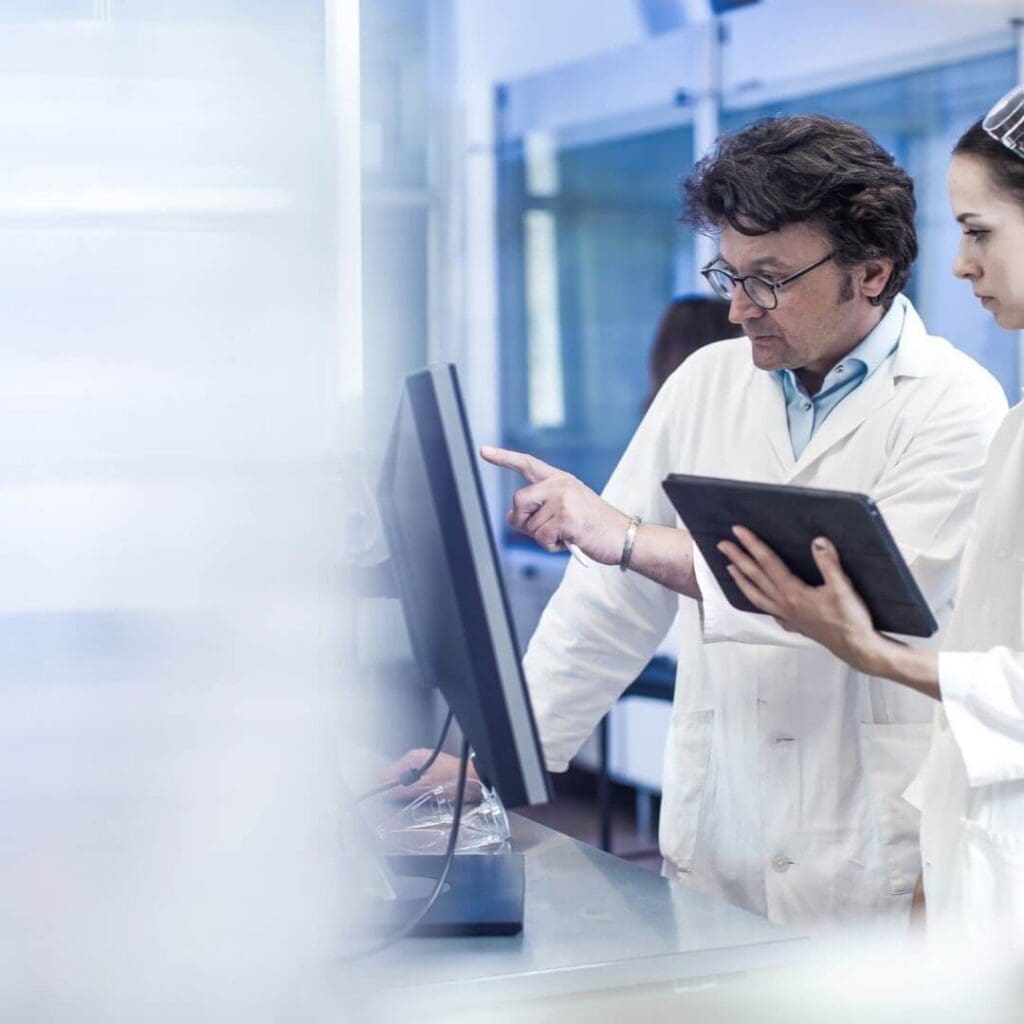
Flexibility is crucial for future-proofed automation solutions. Here are five reasons why
Read more Five reasons flexibility needs to be a standard feature of automation solutions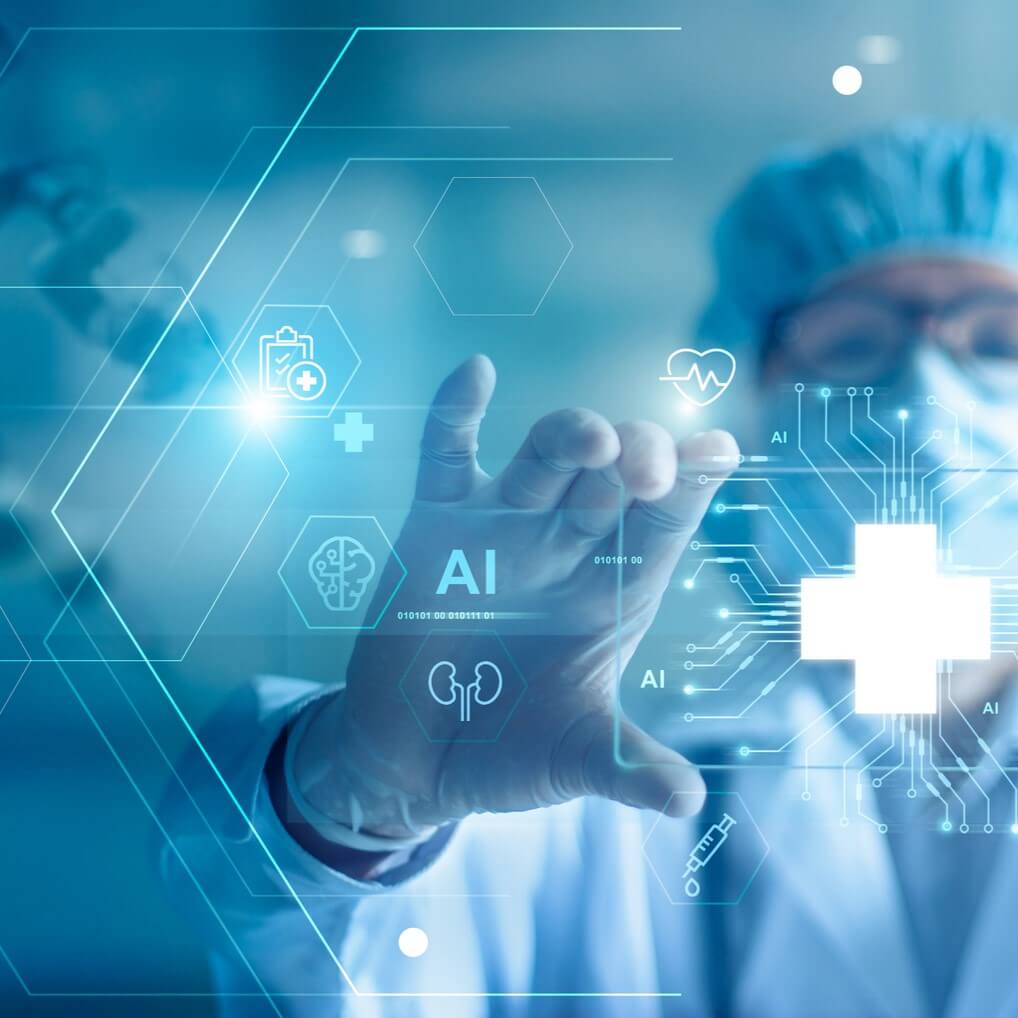
How can lab automation help ensure that the data we generate now…
Read more Data and reproducibility: creating a single source of truth for your lab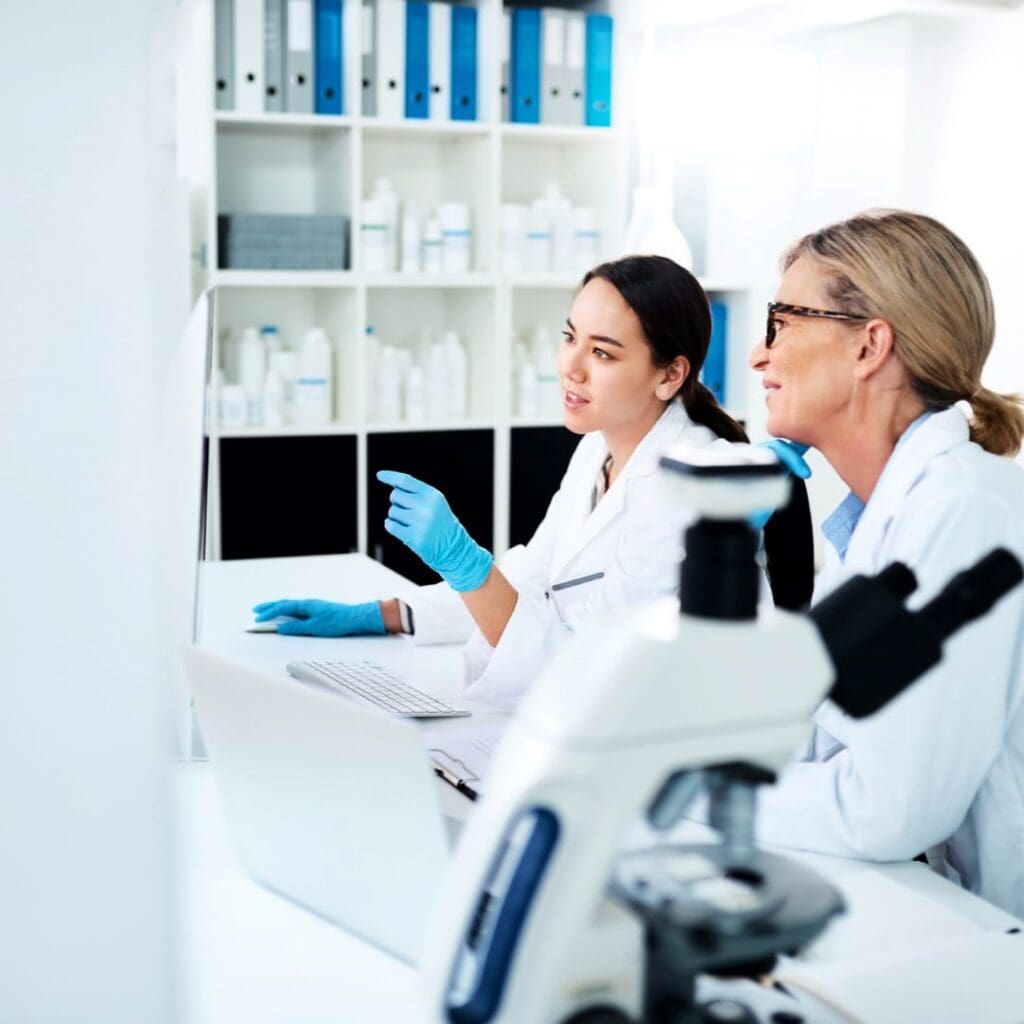
Why is lab automation so hard to use? And why does it…
Read more Balancing usability and functionality