Every day, researchers produce vast amounts of data, from clinical trials, genomic studies, experimental research and more. While this explosion of information holds the potential for groundbreaking discoveries, it also presents a host of challenges.
How do life science professionals manage such massive data volumes? How can they ensure data accuracy, consistency, and accessibility in their quest to solve complex biological problems? The answer lies increasingly in the adoption of automation. Let’s explore.
The challenges of data in life sciences
1. Volume and complexity of data
Life sciences data is vast and diverse. The sheer volume of information is staggering, from genomic sequences to patient health records to proteomics and drug screening results. These datasets are often complex and multidimensional and from various sources. Integrating and interpreting such diverse datasets can be a daunting task. Traditional data management methods fall short, needing help to keep up with the pace and scale of modern research.
2. Data quality and integrity
Maintaining high-quality data is critical in life sciences, where inaccuracies can lead to flawed experiments, incorrect conclusions, and wasted resources. Inconsistent data formats, human errors during data entry, or even equipment calibration issues can compromise data integrity. Ensuring data is accurate, reliable, and standardised across different studies and institutions is an ongoing challenge.
3. Data accessibility and collaboration
Collaboration is critical in the life sciences, as researchers, clinicians, and organisations often need to share and analyse data across geographies and disciplines. However, differing data formats, incompatible systems, and siloed databases frequently hinder this process. Researchers can spend more time wrangling data than actually analysing it, slowing down the pace of discovery.
4. Regulatory compliance and data security
Life sciences data, particularly clinical and patient data, is subject to stringent regulations such as HIPAA, GDPR, and other local laws. Ensuring compliance while managing large datasets adds another layer of complexity. Given the sensitive nature of much of the information, data security is also a major concern.
5. Limited resources and time constraints
Many life science organisations, especially smaller research labs or startups, need more resources dedicated to data management. Hiring specialised data scientists or IT professionals is not always feasible. Meanwhile, the pressure to deliver results quickly—whether for publishing research or advancing a drug through clinical trials—is immense
How automation can help overcome these challenges
Automation offers a powerful solution to many of the challenges outlined above.
1. Streamlining data collection and processing
Automated systems can efficiently handle the vast amounts of data generated in life sciences. Connected automation platforms like LINQ can automate repetitive data entry tasks, reducing errors and freeing up valuable time for researchers. When this kind of clean data is available, AI algorithms can sift through these vast datasets to identify patterns and insights that the human eye might miss.
2. Ensuring data quality and consistency
Standardised data entry processes can be enforced using automation, reducing the risk of human error. With some automation platforms, it’s possible to integrate data collected at experiment level with other sources of information, so if your lab has ML, you can even train it on clean data to predict and correct errors, further enhancing data quality.
3. Improving data integration and accessibility
Automation can simplify the integration of data from multiple sources, whether internal databases or external public datasets. Advanced data integration tools can automatically convert different data formats into a standardised structure, making it easier to share and collaborate. Cloud-based platforms like LINQ are equipped with automation capabilities that allow for seamless, real-time data sharing among researchers across the globe.
4. Facilitating compliance and enhancing security
Automated compliance monitoring tools can help life sciences organisations adhere to regulatory standards by continuously checking data against predefined rules. These tools can automatically generate audit trails and reports required for regulatory submissions, reducing the administrative burden.
5. Maximising efficiency with limited resources
Automation provides a way to do more with less for organisations with limited resources. Automated systems can handle tasks that would otherwise require dedicated personnel, such as data entry, cleaning, and analysis. This enables smaller teams to focus on high-value activities like hypothesis generation, experimental design, and result interpretation. By reducing the manual workload, automation helps accelerate the research cycle, allowing faster discoveries and innovations.
5. Enabling the use of advanced technologies
By making data cleaner, more contextualised and accessible, advanced technologies like artificial intelligence (AI) and machine learning (ML) can be leveraged, transforming how data is used and shared. With open-access automation solutions, AI can draw from automated workflow results for training and subsequent analysis and hypothesis generation, making the possibility of closed-loop, in-silico iteration cycles a reality.
Embracing automation for a data-driven future
While the challenges of data management in life sciences are significant, automation presents a compelling path forward. By leveraging technology to streamline data collection, ensure quality, facilitate collaboration, and maintain compliance, life sciences organisations can focus more on what they do best – advancing our understanding of life and improving human health.
The future of life sciences is undeniably data-driven, and those who embrace automation will be better positioned to navigate the complexities of this evolving landscape, driving innovation faster and more effectively than ever before.
A new kind of automation is tackling these issues
Meet LINQ, the vendor-agnostic, adaptable, error-handling, AI-ready data generating workflow automation platform.
LINQ is composed of two parts – LINQ Bench and LINQ Cloud.
With a customisable configuration, LINQ Bench is designed to fit into any laboratory and accommodate the majority of machinery. Its modular design means that the individual components of the system can be adapted as and when required and control is possible at a local workcell and total workflow level.
LINQ Cloud features workflow building, simulation, validation, execution and control. Workflows can be fully customised with a user-friendly interface, API or SKD, and cloud-based access ensures control over the platform from anywhere in the world. LINQ Cloud also facilitates real time data transfer of fully contextualised workflow results, delivered to a data lake of your choice in an AI-ready format.
The utilisation of LINQ enabled one lab to reduce manual interaction time by 95%, while another increased its throughput by condensing a 6-hour cell culture process into just 70 minutes.
To hear more about what makes LINQ a different kind of automation platform, get in touch with the team today.
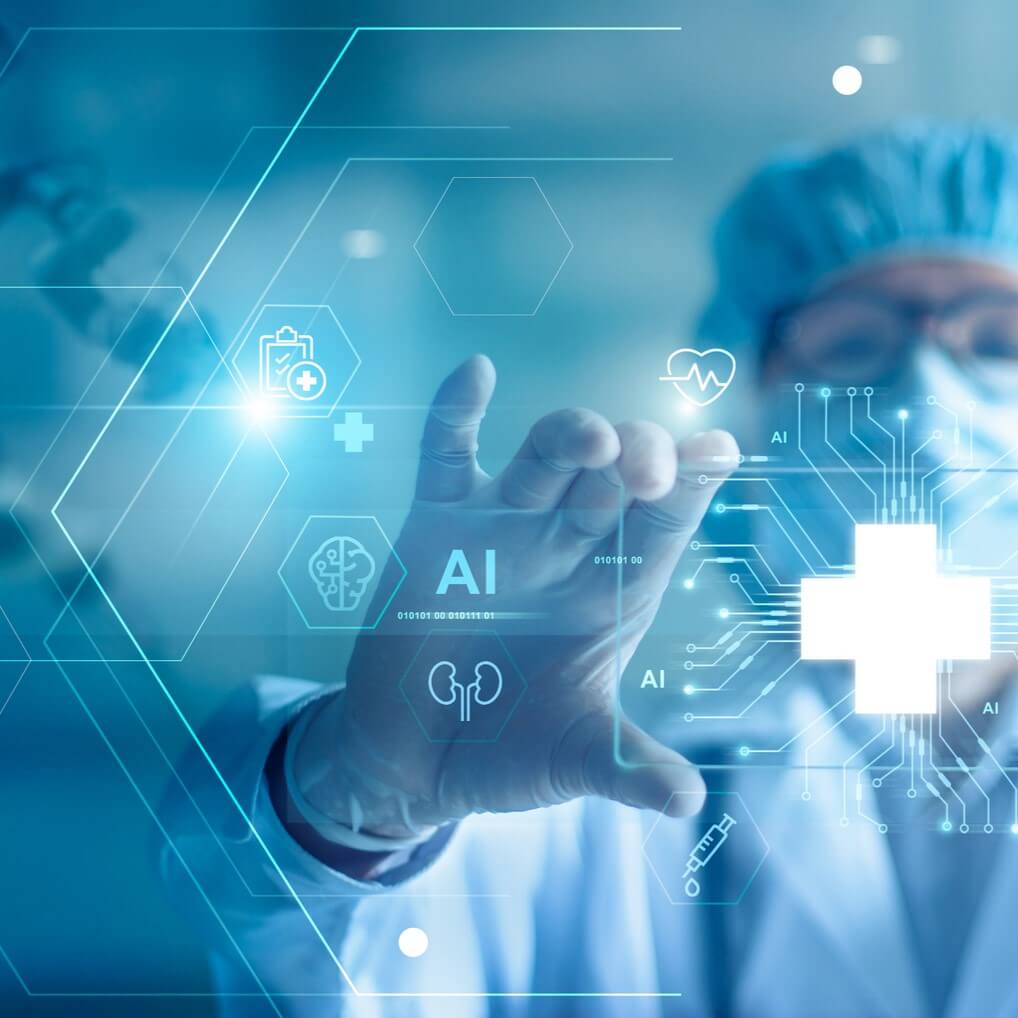
Build Trust in Lab Automation with Reliable Data
Learn how LINQ enables real-time data transfer, traceability, and reproducibility for a single source of truth in your lab.