In this short blog, we explore the value of reproducibility in the lab, and how automation can help teams across the globe collaborate from a single source of reproducible truth.
What is lab reproducibility and why is it important?
Lab reproducibility is being able to repeat research with the same input data and experimental methods and analysis, to achieve results that are consistent with the original findings.2
Scientific discoveries must be reproducible to be trustworthy, but there’s a reproducibility crisis in science according to a survey conducted by Nature in 2016.1
In the survey, over 70% of the 1,576 researchers that were questioned described having tried and failed to reproduce another scientist’s experiment. Whilst a level of uncertainty is inevitable in science, once the statistical analysis has been performed, the results between repetitions should fit within the bounds of the error.
Reproducibility is the key to impactful and collaborative research.
Laboratories across the world can be completely disparate environments, using different experimental procedures and operating conditions. Yet, experiments performed on one side of the globe must be able to be reproduced on the other for us to be truly confident in the conclusions we draw.3
Lab reproducibility vs repeatability
Both reproducibility and repeatability are crucial factors when measuring the quality of experiments.
While reproducibility is the measure of whether results can be attained using different research methods, repeatability speaks to the likelihood of being able to produce the exact same results from the same experiment.
Repeatability helps researchers to verify their results are true and not down to chance. In order to measure repeatability key conditions of the experiment must be kept the same, such as location, apparatus, time and the observer; reproducibility should test these methods to reinforce the results, and to assure against human error or fraud.
How do you evaluate the reproducibility of an experiment?
In order to gain real reproducibility, the reporting of the experiment is critical. Experiments can be assessed at an individual, project, lab, or even field level, but reproducing the same results under varied conditions is the key to deciding whether something is or isn’t reproducible.
The book The Practice of Reproducible Research6 suggests three ways reproducibility can be assessed at a research project level; automation and provenance tracking, availability of software and data, and open reporting of results.
Automation and provenance in assessing reproducibility
Automation enhances the repeatability of a project in a number of ways explored above, however, it’s also important to assess the automation used and how it can support the overall assessment of experiment reproducibility.
For example, automation that requires extensive manual set-up at the start of an experiment may be less useful in supporting reproducibility because it still involves manual interventions.
Similarly, automation that doesn’t consider the tracking of data, from raw to analysis to calculations also doesn’t support reproducibility in the same way as automation that provides audit trails.
One of the key benefits of automating with LINQ lies in its ability to handle data, and to make it more useable.
LINQ can send real-time results from each point in your workflow to your LIMs for instant assessment, and it provides a robust audit trail so you can see exactly what is happening at each stage of your experiment. This can be shared and examined by others at any location, and easily duplicated for repeatability and reproducibility assessment.
One CRO we worked with wanted to scale their virology ELISA testing capabilities, so chose to automate due to its ability to reduce contamination risks, while improving the quality of results and the data collected. Our LINQ Cloud laboratory orchestrator was used to provide a software platform that seamlessly connected each activity in the ELISA workflow, providing full traceability for each sample, and adding test data to the lab’s LIMS. Barcode scanners on each LINQ module and within the instruments themselves provided tracking for each sample, right down to its position within each microplate. As a result, this CRO was able to confidently reproduce experiments for its clients while reaping all the other benefits automation has to offer.
Availability of software and data in assessing reproducibility
In order to make research widely reproducible, experimental data and the software used to gather or record it must be available.
Consider, for example, whether web-based sharing has long-term viability; whether the data is in a commonly used format; if the software used is readily available; and how well the software itself has been tested and documented.
Open reporting of results in reproducibility
Studies are considered reproducible when sufficient details about their execution are available, so open reporting is a key measure when assessing reproducibility. This is why most results aim to be published in a public setting, for example in a journal or at a conference.
Research may be published in either green access journals – those that typically charge a subscription/access fee for their content – or gold access journals, those that have freely available content, where a fee may be paid by the author rather than the reader.
Both are viable ways to openly report results to support credible reproducibility, and there is evidence that publication in open-access forums promotes the use of scientific findings, hence, further providing true reproducibility.
How do you ensure the reproducibility of results?
To ensure reproducibility in research, we need to strictly adhere to basic scientific principles. For example:
- Optimising robust experimental protocols
Over time, experimental procedures used by different members of the same lab can diverge. Whilst these differences can be subtle, the effects can be huge, causing large discrepancies in results. Optimising protocols for synchronicity between researchers is essential for reproducibility. This can be ensured by preparing standard operating procedures (SOPs) to be followed by all users, which in turn can easily be widely distributed to researchers in other lab groups. - Publication of all data, statistical analysis, and full results
Publishing meaningful results through a process of rigorous peer review is fundamental to the scientific method. However, papers are often published without disclosing all of their data, complete methods of analysis, or full results, including the negative or confusing. To ensure reproducibility in the lab, transparency is key. We must endeavour to publish everything so that other scientists can properly review our work. - Automation of lab processes
Typically in the lab, human researchers undertake the experiments. Fundamentally, it is a well-known fact that humans make errors, no matter how careful they may try to be. And, as mentioned above, experimental protocols can subtly vary from researcher to researcher. Automation of common lab processes can reduce human error, and prevent variation between experiment repetitions, thus improving the reproducibility and reliability of experimental results.
The impact of automation on lab reproducibility
Automation in the lab can significantly enhance the reproducibility of experiment results, even between separate laboratories across the globe. A typical day in the lab often involves many repetitive, tedious tasks that are common throughout research, such as aliquoting, centrifugation or sample sorting.
It is these monotonous processes that are the most prone to human error, and it is precisely these tasks that lab automation can impact. Automating routine techniques forgoes the risk of subtle protocol divergence between researchers, and increases lab productivity.4
The quality of experimental protocol and output results can be improved through automation. The ability to programme automated processes in the lab allows for the precise operation of experiments. Protocols can more easily be shared and distributed between researchers, enabling standardisation of experiments and improved quality control.
Automation allows for faster, more efficiently run experiments. Whilst humans tire, lab robots do not, thus high throughput screening and analysis of large data sets provide improved reliability of the output results.5 Therefore, we can ensure the reproducibility of experimental results, supporting scientific discovery and innovation by providing confidence to researchers in the outputs they obtain in the lab.
Automation solutions from Automata
Automata LINQ is a lab automation platform that enables life sciences labs to fully automate their workflows.
We do this with our automated lab bench, which fits into the same footprint as a regular lab bench, and lab orchestration software, which enables true walkaway time.
References
1. Baker, M. 1,500 scientists lift the lid on reproducibility. Nature 533, 452–454 (2016).
2. Ensuring Reproducible Data in the Laboratory. Lab Manager https://www.labmanager.com/business-management/ensuring-reproducible-data-in-the-laboratory-23958.
3. Reproducibility: 8 steps to make your results reproducible. https://blog.genofab.com/increase-reproducibility.
4. Holland, I. & Davies, J. A. Automation in the Life Science Research Laboratory. Frontiers in Bioengineering and Biotechnology 8, (2020).
5. Jessop-Fabre, M. M. & Sonnenschein, N. Improving Reproducibility in Synthetic Biology. Frontiers in Bioengineering and Biotechnology7, (2019).
6. Kitzes, J., Turek, D., & Deniz, F. (Eds.). (2018). The Practice of Reproducible Research: Case Studies and Lessons from the Data-Intensive Sciences. Oakland, CA: University of California Press.
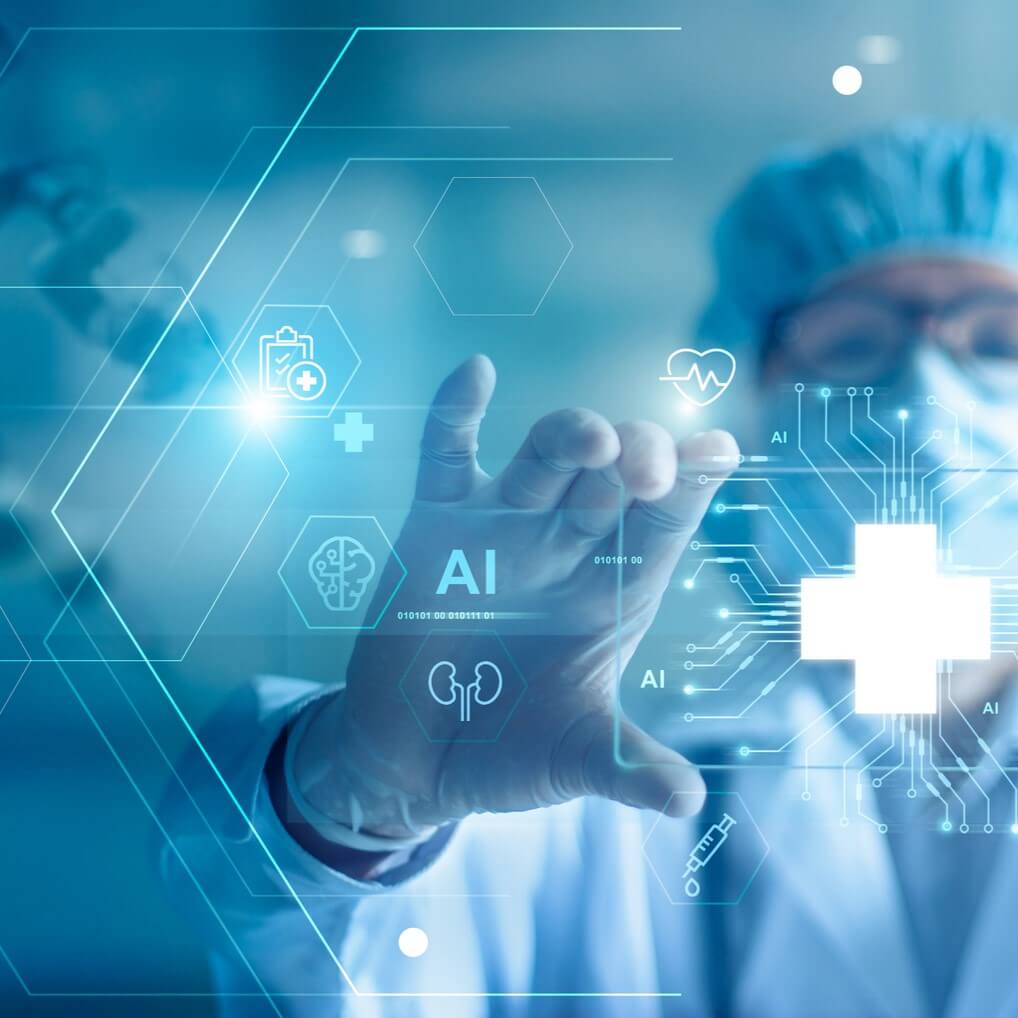
How can lab automation help ensure that the data we generate now…
Read more Data & Reproducibility: Single Source Of Truth In The Lab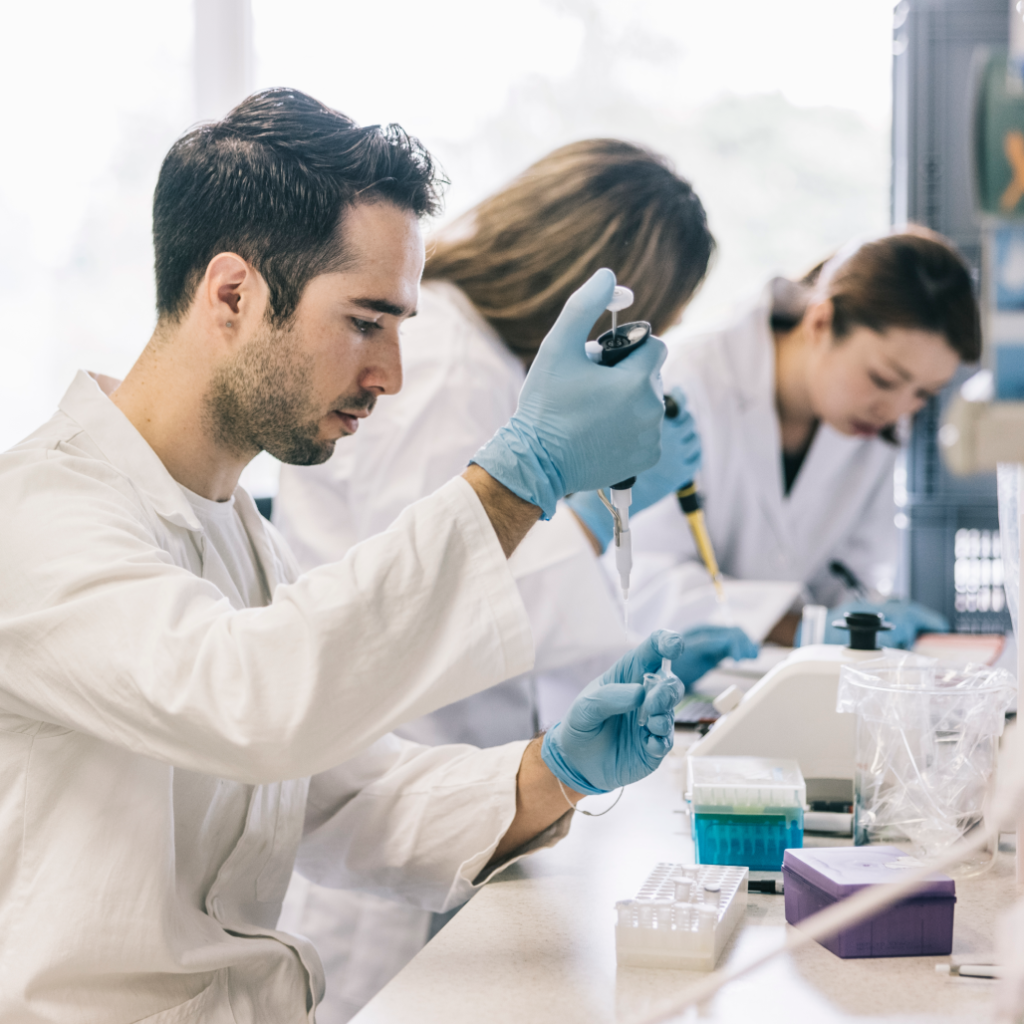
We look at what causes errors in biology lab experiments and how…
Read more Common sources of error in biology lab experiments